05.22.2024 • Thomas Castermans, Chief of Staff
GenAI: An emerging concern in the enterprise data privacy realm
As enterprises worldwide embrace the transformative power of GenAI, safeguarding data integrity is becoming paramount for navigating the evolving landscape of privacy and innovation.
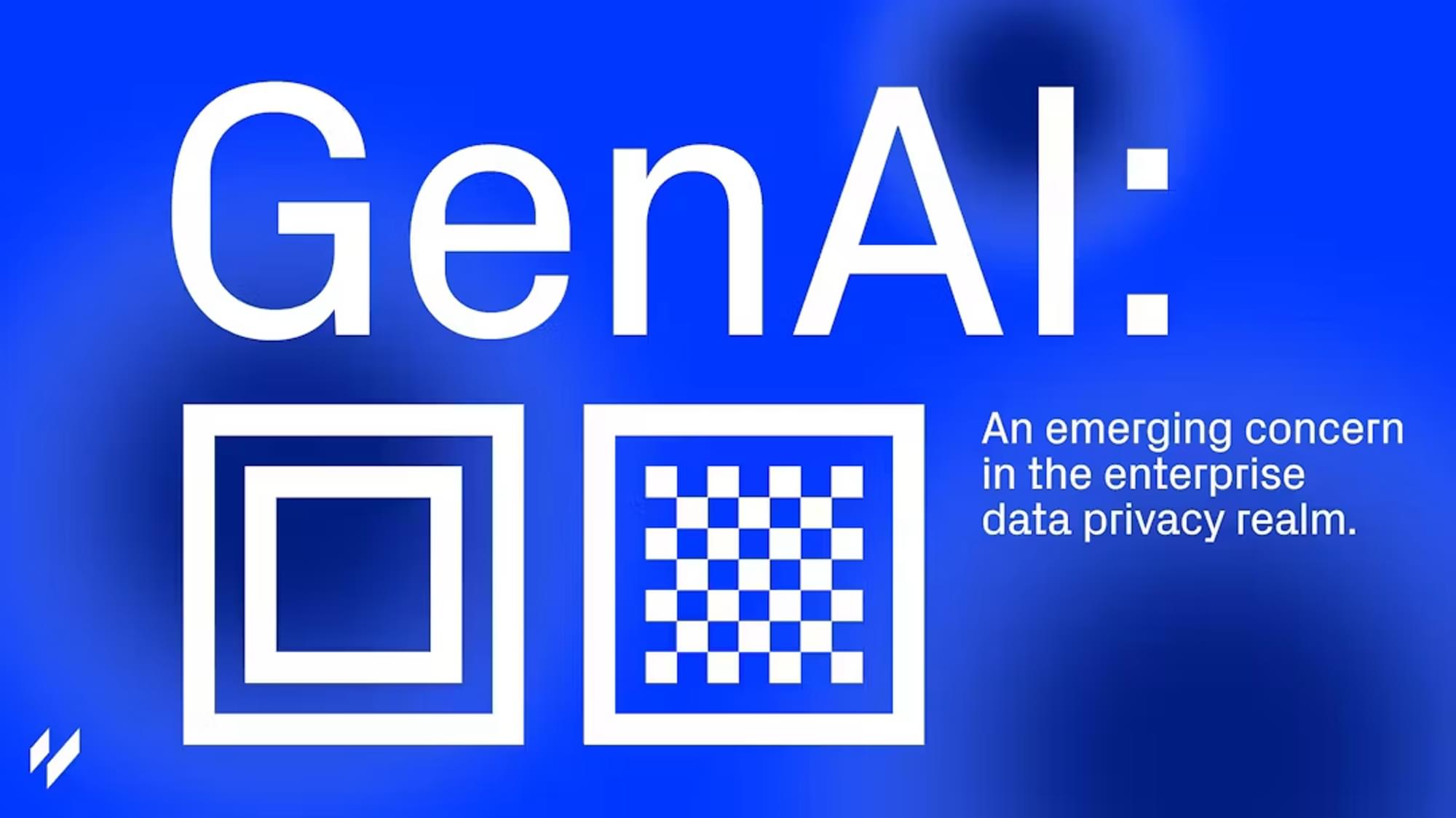
Last year, generative artificial intelligence (GenAI) emerged prominently, capturing widespread attention, fueling a surge of dedicated startups, and prompting many small- and medium-sized enterprises (SMEs) and multinational corporations to reshape their strategic roadmaps. Deloitte predicts that in 2024, enterprise spending on GenAI will grow by 30%, from an estimated US$16 billion in 2023.
The integration of innovative GenAI tools into enterprise operational processes launched a new era characterised by unparalleled creativity, functionality and efficiency. Specifically, the adoption of large language models (LLMs) such as Copilot, Lambda, and Falcon 40B has prompted organisations to swiftly develop and deploy GenAI-driven applications and services tailored to their diverse industry needs. This trend has significantly impacted conventional approaches to digital transformation. However, to make the most out of enterprise GenAI applications, organisations must consistently and securely incorporate extensive datasets into their machine learning (ML) models. Ultimately, the quality of algorithms’ output hinges on the quality of data employed to train them.
Although GenAI shows immense potential, the security and privacy risks linked to the ingestion and exposure of sensitive data, such as personally identifiable information (PII) and protected health information (PHI), cannot be overlooked. This has sparked concerns among business executives striving to safeguard their data against unauthorised disclosure, breaches and leaks.
What is the current sentiment towards GenAI in the enterprise world?
Cisco’s 2024 Data Privacy Benchmark Study, an annual evaluation of critical privacy concerns and their business ramifications, analysed the escalating privacy challenges associated with GenAI confronting organisations. Based on feedback from 2,600 privacy and security experts spanning 12 regions, the seventh edition of the study underscored that privacy transcends mere regulatory compliance. Among the key concerns, businesses highlighted the threats to an organisation’s legal and intellectual property rights (69%), as well as the risk of disclosure of information to the public or competitors (68%).
While most organisations acknowledge these risks and are implementing measures to mitigate exposure — with 63% establishing restrictions on data input, 61% imposing limits on employee access to GenAI tools, and 27% temporarily prohibiting GenAI applications altogether — a significant portion of individuals continue to input problematic data into GenAI tools, including employee information (45%) and non-public company data (48%).
What are the key enterprise concerns with GenAI?
It is crucial to recognise that ML models are dynamic systems that continuously evolve based on the data they ingest and process, learning and adapting to diverse datasets. This adaptiveness introduces inherent security risks that organisations must approach with caution.
Models like ChatGPT, which train on extensive general knowledge datasets, often sourced from platforms such as Wikipedia, face the risk of inadvertently incorporating “poisoned” data. Likewise, businesses train their ML models with datasets sourced internally or aggregated from third-party sources. There's a potential danger that these datasets might harbour hidden and unidentified malware, similar to ransomware, aimed at compromising system integrity. If the training data includes misinformation or malicious content, it becomes integrated into the ML model's learning process. Needless to say, even a minor presence of “poisoned” data has the potential to rapidly escalate into a significant issue, impacting operational continuity.
Another concern involves the incorporation of PII into the training datasets. While “poisoned” data often brings to mind images of malware, threats and immediate dangers, its implications stretch further to encompass safety hazards. The inclusion of PII in the data repositories utilised for training ML models can result in the misuse of personal data, presenting substantial risks to both individuals and organisations, among which accidental data loss and disclosure and privacy violations.
How can businesses minimise existing risks?
As businesses explore the transformative capabilities of GenAI, particular applications and services are significantly shifting the course of enterprise digital transformation efforts. Nonetheless, these initiatives present distinct challenges, particularly concerning the security, protection and privacy of sensitive enterprise data.
Training models requires gathering and organising extensive amounts of unstructured data, which is crucial for the model's effectiveness. At Valarian, we believe that this process needs to be safeguarded to prevent any instances of unauthorised data exposure and breaches of data privacy. Companies embracing GenAI should incorporate privacy by design to proactively streamline knowledge handling, boost operational effectiveness, and guarantee the secure and ethical application of innovative tools.
Protecting PII before it's integrated into an LLM is akin to safeguarding data against malware before it reaches an organisation's endpoint. Both measures aim to mitigate significant issues and potential liabilities before they emerge. All this to say that the rise of AI advancements further emphasises the necessity for proactive enterprise data security measures and strategies.
What does the future hold?
The swift adoption of GenAI has introduced new opportunities for organisations aiming to leverage its transformative capabilities, along with numerous challenges and risks that have the potential to hinder data security and privacy. The urgency of ensuring the security of data ingested by the ML models is emphasised by their dynamic characteristics, highlighting the need for robust privacy protection methods. Effective GenAI integration, therefore, requires a robust data security strategy, prioritising the protection of sensitive data and minimising the risk of privacy breaches.
To navigate this dynamic landscape, organisations must foster ongoing innovation and embrace technologies that can empower them to uphold security and adhere to regulatory requirements. Valarian’s platform for privileged enterprise communication is an example of a tool that ensures data privacy and control, safeguarding any sensitive information that is being exchanged from invasive data harvesting practices. By giving equal importance to privacy, security and innovation, organisations can unlock the complete potential of GenAI while preserving the trust of users and stakeholders.